The Impact of Machine Learning on Payroll Fraud Detection
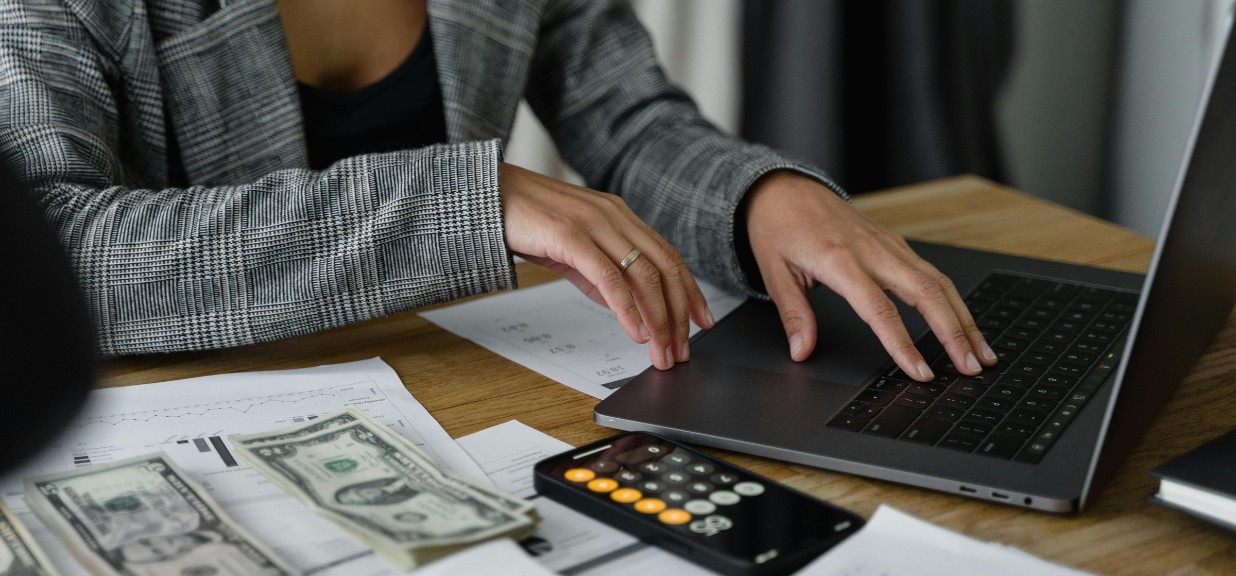
Are you familiar with 'The Phantom Workforce Incident'?
The incident occurred when a departmental payroll manager from a leading retail firm created several ghost employees over the years, diverting their alleged wages into an account controlled by fraudsters. This wrung millions of dollars from the company, causing the company to incur huge financial losses.
Payroll fraud cases like this can showcase the impact on an organization's operations, reputation, and employee morale. The payroll fraud punishment for such crimes can be severe. Payroll fraud cases like this can showcase the impact on an organization's operations, reputation, and employee morale.
So, the question arises: How can organizations protect their payroll programs and preserve the valuable trust in their workforce from payroll fraud? This challenge is particularly acute as payroll fraud is difficult to detect and complex due to its causes and nature. Leveraging Machine Learning (ML) can lead to powerful methods to improve the performance and quality of payroll processing by designing new models to anticipate fraud. This brings great opportunities for payroll processors, protection, and fraud detection specialists.
What does Payroll Fraud mean?
Payroll fraud is a complex problem because it is multifaceted. It harms organizations financially and commoditizes the trust that forms the bedrock of successful business exchanges. Each form of payroll fraud poses unique risks.
Recognizing the different payroll fraud schemes is crucial to alert organizations about potential vulnerabilities that can impact the legitimacy of payroll records. Exposing the mechanics of ghost employees, timesheet fraud, and misclassification can help detect and combat payroll fraud. Vigilance, intelligent detection mechanisms, and understanding the risks of payroll fraud are also crucial for payroll professionals to safeguard the integrity of payroll data.
- Ghost Employees: This refers to creating imaginary workers and adding them to the company payroll, depleting the firm's resources without performing any work.
- Timesheet Fraud: It occurs when employees inflate their working hours or claim payment for hours not worked, leading to unjustified payroll expenses.
- Misclassification: Workers may be incorrectly categorized to avoid paying overtime or providing benefits, contravening labor laws, and affecting employee rights.
The Transformative Power of Machine Learning
As the business landscape constantly evolves, keeping up with fraudsters' strategies in fast-paced work environments is becoming increasingly challenging. This is why Machine Learning has the potential to take on payroll fraud.
By leaning on ML algorithms' strong pattern recognition and predictive benefits, companies can stay ahead of payroll fraud and potentially even stop all threats before they ever happen.
Investments in technologies for fraud prevention are a part of this financial landscape, highlighting the importance of efficient resource allocation to safeguard a company's assets and ensure its growth. Effective financial strategies enable organizations to allocate resources wisely across all departments, including payroll, to combat fraud with advanced technological tools.
You might also like | Revolutionizing Payroll with AI and Machine Learning
- Pattern recognition: ML algorithms are particularly good at finding patterns and anomalies in datasets. This allows companies to identify oddities in payroll transactions that could indicate fraud.
- Anomaly detection: Look for anomalies in the data. For example, anomaly detection algorithms could identify outliers in payroll amounts or timesheet entries that deviate from the usual patterns, potentially signaling fraud.
- Predictive Analysis: These cases also illustrate the variety of tricks swindlers could employ and the need to watch for payroll duties.
Spotlight on ML Models
Each ML model has specific characteristics that better contribute to paying attention to different signals in the dataset. Some of the models that can be implemented in payroll systems include:
- Logistic Regression: This model helps identify specific irregularities, such as unusual payment amounts or patterns that deviate from the norm.
- Isolation Forests: These models can detect anomalies from dataflows that can help detect odd payroll transactions that could indicate errors within the company or internal fraud.
- Neural Networks: Neural networks can detect more sophisticated fraud schemes than simpler models, as they can learn low-level features in payroll data.
Enhancing Detection: Use of Advanced Analytics
Analytics infused with predictive capabilities helps fight payroll fraud by foreseeing relative likelihoods of fraud. Predictive Analytics uses historical data to forecast future events, providing a predictive edge in identifying potential payroll fraud before it occurs.
Additionally, layering predictive analytics with blockchain to combat payroll fraud can help organizations stay one step ahead of fraudsters to protect their assets from financial losses and organizational reputation.
How do you prevent Payroll Fraud?
While machine learning is a powerful tool against payroll fraud, human expertise remains indispensable for interpreting data anomalies, ensuring ethical use of technology, and continuously adapting strategies as new fraud tactics emerge. A hybrid approach leveraging both technological and human intelligence is most effective. Experts provide context to data patterns identified by machine learning, guarding against false positives and exploring root causes. Their ethical oversight protects employee privacy and prevents algorithmic bias. As fraudsters evolve tactics, human practitioners train machine learning models to evolve in parallel. The use of AI in payroll fraud detection is becoming increasingly important.
Also Read | Chatbots, Machine Learning & Beyond: Enhancing Employee Experience with AI
However, an organizational culture of transparency and accountability is the critical foundation. Such a culture deters would-be fraudsters while empowering a vigilant workforce to spot vulnerabilities before issues arise. Transparent processes, strong accountability standards, and open communication channels promote ethical conduct aligning with organizational values. This cultural bedrock complements the technological capabilities of machine learning and blockchain's tamper-proof recordkeeping.
Ultimately, a multifaceted strategy integrating machine learning's predictive analytics, blockchain's immutable audit trails, human contextual judgment, and an organizational culture of integrity is paramount. Technology powers robust detection, but human insight interprets findings ethically, while corporate culture disincentivizes fraud attempts. This holistic approach protects finances, maintains employee trust and morale, and future-proofs payroll defence as threats evolve.
Leave no room for error, by joining Neeyamo’s global payroll revolution. Neeyamo’s Global Payroll solution ensures vigilant security measures including the use of ML, acting as a vault for payroll data. Reach out to our experts at irene.jones@neeyamo.com.
Latest Resources
Stay informed with latest updates
If you're curious and have a thirst for knowledge pertaining to the HR, payroll, and EOR universe, don't miss out on subscribing to our resources.